AI for Science Seminar Series (Spring 2024)
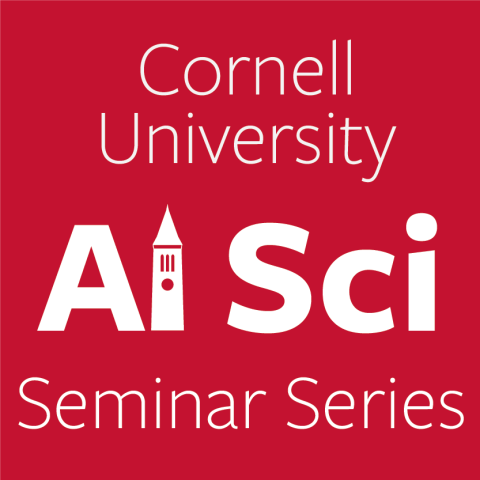
We are excited to announce the AI for Science seminar series which aims to bring together the computational and scientific communities to understand advances and challenges at the frontier of AI for scientific discovery. The seminars will feature both pioneers and Schmidt Sciences AI in Science Postdocs from nine participating institutions.
2:30–3:30pm alternating Fridays
February 9–May 3, 2024
Gates 122 and via Zoom
Our inaugural speaker will be Prof. Stefano Ermon on applications of diffusion models to multiple areas of science, including materials discovery and climate science. Recordings will be made available on our YouTube channel.
If you have speaker suggestions or are a Schmidt Sciences AI in Science Postdoc who is interested in presenting your research, please contact Laura Greenstreet.
Spring 2024 Seminars
Date/Time | Speaker | Title | Links |
---|---|---|---|
Fri Feb 9, 2024 2:30–3:30pm EST (UTC-5) | Prof. Stefano Ermon, Stanford University | Diffusion Models for Scientific Discovery | |
Fri Mar 15, 2024 2:30–3:30pm EDT (UTC-4) | Dr. Fatema Tuz Zohora, University of Toronto | Unraveling Cancer Cell Communication Using Deep Learning Models on Spatial Transcriptomics Data | |
Dr. Xiaoyu Zhao, UCSD | AI identifies Tumor Protein Complexes to Predict Chemoresistance | ||
Fri Mar 22, 2024 2:30–3:30pm EDT (UTC-4) | Prof. Michael Bronstein, University of Oxford | A Physical Perspective on Graph Neural Networks | |
Fri Apr 12, 2024 2:30–3:30pm EDT (UTC-4) | Prof. Aditya Grover, UCLA | Foundation Models for a Sustainable Planet | |
Fri May 3, 2024 2:30–3:30pm EDT (UTC-4) | Prof. Kyunghyun Cho, New York University | Machine Learning in Prescient Design's Lab-in-the-Loop Antibody Design |
Spring 2024 Seminar Details
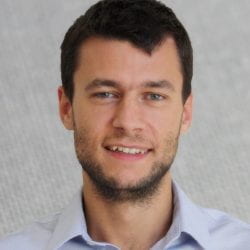
Prof. Stefano Ermon, Stanford University
Fri Feb 9, 2024, 2:30–3:30pm EST (UTC-5)
video
Title: Diffusion Models for Scientific Discovery
Abstract: Diffusion models are at the core of many state-of-the-art generative AI systems for media content such as images, videos, and audio. Due to their excellent sample quality and theoretical guarantees, they are emerging as an important tool in many scientific, medical, and engineering applications. In this talk I will present several extensions of diffusion models tailored to the unique challenges that arise in these domains. I will discuss techniques for incorporating prior knowledge through constraints, symmetries and invariances for geometric data (such as molecules), and new approaches for tackling inverse problems. These techniques provide significant benefits across a variety of applications ranging from molecule generation to medical imaging.
Bio: Stefano Ermon is an Associate Professor in Stanford’s Department of Computer Science and a senior fellow at the Woods Institute of the Environment. His research focuses on machine learning and generative AI motivated by real-world applications in science and engineering with societal relevance. Dr. Ermon has received many awards including ONR and AFOSR Young Investigator Awards, Sloan and Microsoft Research Fellowships, and an NSF CAREER award and is a co-founder of Atlas AI. He received his PhD from Cornell University working with Cornell University AI for Science Institute co-director Carla Gomes.
AI for Cancer Research
Fri Mar 15, 2024, 2:30–3:30pm EDT (UTC-4)
video
This is the first of our seminars featuring postdocs from The Eric and Wendy Schmidt AI in Science Postdoctoral Fellowship program. Through the program, over 160 postdoctoral fellows from 9 universities use AI to accelerate research on the frontier of natural sciences, engineering, and mathematical science.
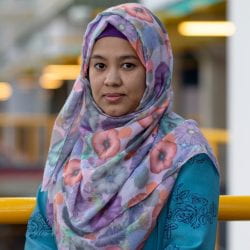
Dr. Fatema Tuz Zohora, University of Toronto
Fri Mar 15, 2024, 2:30–3:30pm EDT (UTC-4)
video
Title: Unraveling Cancer Cell Communication Using Deep Learning Models on Spatial Transcriptomics Data
Abstract: Cells coordinate within tissue to carry out complex functions, but dysregulation of communication between cells may lead to diseases such as cancer and diabetes. Identifying cell-cell communication that is upregulated in disease can lead to the development of better therapeutics. Existing computational methods for predicting communication in tumor samples often involve experiments that collect a single molecular layer from individual cells and do not take the spatial location of the cells into account. As a result, most existing methods exhibit high false positive rates. Moreover, most tools predict communication between cell populations instead of detecting communication at single-cell resolution, which misses the correlation between cellular communication and cancer heterogeneity. In this talk, I will present ‘NEST: NEural network on spatial transcriptomics’, to address these limitations. NEST uses a graph attention network paired with an unsupervised contrastive learning approach to detect cellular communication while retaining the strength of each signal. NEST outperforms other methods on synthetic benchmarking experiments and detects biologically-relevant communication along with directionality and confidence across spot- and cell-based spatial transcriptomic technologies, tissues, and diseases. NEST identifies region-specific T-cell homing signals in human lymph nodes, aggressive cancer communication in lung adenocarcinoma, and discovers new communication patterns in pancreatic cancer. NEST is not limited to a single technology or species. Instead, it is a transferable model applicable to data across domains. We believe NEST makes a notable contribution to accelerating the application of deep learning in single-cell research and other related knowledge-graph based contexts.
Bio: Fatema Tuz Zohora is a Schmidt AI in Science Postdoctoral Research Fellow at Princess Margaret Cancer Centre, University Health Network, University of Toronto, Canada. She received her PhD in Computer Science from the University of Waterloo, Canada. Prior to that, she served as an Assistant Professor at Bangladesh University of Engineering and Technology, Bangladesh. She is experienced in the research and development of deep learning models that are applicable to Knowledge Graphs, Computer Vision, and Natural Language Processing problems. She has applied her expertise in Single-cell Analysis, Proteomics, Genomics, and clinical data analysis.
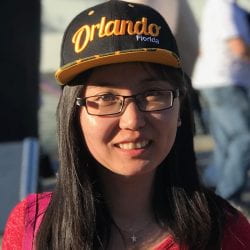
Dr. Xiaoyu Zhao, UCSD
Fri Mar 15, 2024, 2:30–3:30pm EDT (UTC-4)
video
Title: AI identifies Tumor Protein Complexes to Predict Chemoresistance
Abstract: Understanding therapeutic resistance in cancer remains a longstanding challenge. Zhao and colleagues construct an interpretable AI system that integrates genetic alterations across molecular assemblies, enabling a quantitative and precise assessment of a tumor’s response to common chemotherapeutics that induce replication stress. The AI implicates 41 molecular assemblies involved in transcription, DNA repair, and cell-cycle regulation which underlie accurate drug response prediction. Thirty of these assemblies, which the authors further explore through CRISPR screenings, are found to regulate drug sensitivity and replication restart. The model translates to cisplatin-treated cervical cancer patients, revealing an RTK-JAK-STAT assembly linked to resistance. This research illuminates how a complex collection of mutations give rise to drug response, offering a valuable tool of interest for precision medicine.
Bio: Xiaoyu Zhao completed her PhD in cancer genomics at Stony Brook University. As a postdoctoral scholar in the Department of Medicine at UC San Diego, she is deeply interested in understanding the complex biological systems and human diseases by integrating genome editing technologies and artificial intelligence (AI) models. Her current focus is on developing deep learning models aimed at improving variant interpretation in the context of drug responses and disease progression.
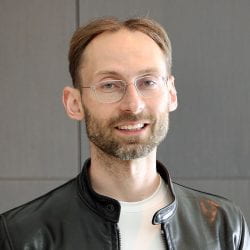
Prof. Michael Bronstein, University of Oxford
Fri Mar 22, 2024, 2:30–3:30pm EDT (UTC-4)
video
Title: A Physical Perspective on Graph Neural Networks
Abstract: The message-passing paradigm has been the “battle horse” of deep learning on graphs for several years, making graph neural networks a big success in a wide range of applications, from particle physics to protein design. From a theoretical viewpoint, it established the link to the Weisfeiler-Lehman hierarchy, allowing to analyse the expressive power of GNNs. We argue that the very “node-and-edge”-centric mindset of current graph deep learning schemes may hinder future progress in the field. As an alternative, we propose physics-inspired “continuous” learning models that open up a new trove of tools from the fields of differential geometry, algebraic topology, and differential equations so far largely unexplored in graph ML.
Bio: Michael Bronstein is the DeepMind Professor of AI at the University of Oxford. He was previously Head of Graph Learning Research at Twitter, a professor at Imperial College London, and held visiting appointments at Stanford, MIT, and Harvard. His research interests include geometric deep learning and shape analysis with applications including molecular and protein design. He has received numerous awards including the EPSRC Turing AI World Leading Research Fellowship, Royal Society Wolfson Research Merit Award, two Google Faculty Research Awards, and two Amazon AWS ML Research Awards. Michael received his PhD from the Technion in 2007. In addition to his academic career, Michael is a serial entrepreneur and founder of multiple startup companies, including Novafora, Invision, Videocites, and Fabula AI.
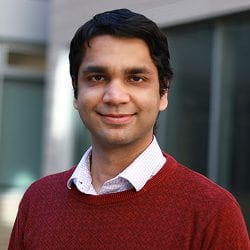
Prof. Aditya Grover, UCLA
Fri Apr 12, 2024, 2:30–3:30pm EDT (UTC-4)
Room Change: Gates G01
video
Title: Foundation Models for a Sustainable Planet
Abstract: Key global sustainability challenges, ranging from ensuring food and energy security to managing disaster response, critically depend on our ability to accurately forecast weather and project climate. While current approaches are limited by our physical understanding of the atmosphere, improvements in sensory capabilities and large-scale machine learning (ML) present immense opportunities for designing alternative solutions. In this talk, I will discuss key design principles for developing foundation models for atmospheric sciences. Unlike language and vision, scientific domains present unique challenges due to the significant heterogeneity of available datasets. Moreover, downstream tasks in atmospheric sciences require generalizing across a broad range of variables and spatiotemporal resolutions. To address these challenges, I will demonstrate novel strategies for data engineering, model design, spatiotemporal optimization, and cross-modal finetuning aimed at scalable learning of atmospheric foundation models. Our resulting models exhibit exceptional skill, speed, and adaptability across a range of predictive tasks in weather and climate science. Finally, I will summarize the broader implications of this research for scientific ML and growing external efforts to adapt our models for diverse sustainable development goals.
Bio: Aditya Grover is an assistant professor of computer science at UCLA. His research interests are at the intersection of generative modeling and sequential decision making, and grounded in applications for accelerating science and sustainability. Aditya’s research has been recognized with a best paper award (NeurIPS), several graduate fellowships and faculty awards (Google, Meta, Microsoft, Schmidt Sciences, Simons Institute), the Forbes 30 Under 30 List, the AI Researcher of the Year Award by Samsung, the Kavli Fellowship by the US National Academy of Sciences, and the ACM SIGKDD Doctoral Dissertation Award. Aditya received his postdoctoral training at UC Berkeley, PhD from Stanford, and bachelors from IIT Delhi, all in computer science.
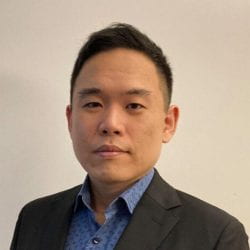
Prof. Kyunghyun Cho, New York University
Fri May 3, 2024, 2:30–3:30pm EDT (UTC-4)
Room Change: Gates G01
video
Title: Machine Learning in Prescient Design’s Lab-in-the-Loop Antibody Design
Abstract: In this talk, I will describe machine learning paradigms that form a foundation on top of which we have built the lab-in-the-loop antibody design platform at Prescient Design, a Genentech Accelerator. These paradigms include multiobjective blackbox optimization and generative models.
Bio: Kyunghyun Cho is a professor of computer science and data science at New York University and a senior director of frontier research at the Prescient Design team within Genentech Research & Early Development (gRED). He is also a CIFAR Fellow of Learning in Machines & Brains and an Associate Member of the National Academy of Engineering of Korea. He served as a (co-)Program Chair of ICLR 2020, NeurIPS 2022 and ICML 2022. He is also a founding co-Editor-in-Chief of the Transactions on Machine Learning Research (TMLR). He was a research scientist at Facebook AI Research from June 2017 to May 2020 and a postdoctoral fellow at University of Montreal until Summer 2015 under the supervision of Prof. Yoshua Bengio, after receiving MSc and PhD degrees from Aalto University April 2011 and April 2014, respectively, under the supervision of Prof. Juha Karhunen, Dr. Tapani Raiko and Dr. Alexander Ilin. He received the Samsung Ho-Am Prize in Engineering in 2021. He tries his best to find a balance among machine learning, natural language processing, and life, but almost always fails to do so.